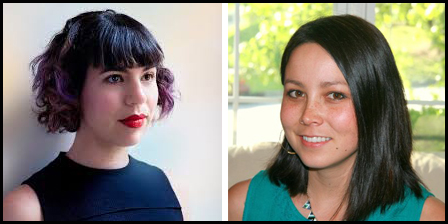
VIDEO OF THIS PANEL (with Special Guest Chelsea Manning!):
Technology Track – Ethical Algorithms – Saturday, November 4th
2:00 – 2:45 pm – Ethical Algorithms Panel – w/Q and A.
Kristian Lum (Human Rights Data Analysis Group – HRDAG) As the Lead Statistician at HRDAG, Kristian’s research focus has been on furthering HRDAG’s statistical methodology (population estimation or multiple systems estimation—with a particular emphasis on Bayesian methods and model averaging).
Caroline Sinders (Wikimedia Foundation) – Caroline uses machine learning to address online harassment at Wikimedia, and before that, she helped design and market IBM’s Watson. Caroline was also just named as one of Forbes’ “8 AI Designers You Need to Know.” Plus Special surprise guest!
About the Ethical Algorithms Panel and Technology Track
by Lisa Rein, Co-founder, Aaron Swartz Day
I created this track based on my phone conversations with Chelsea Manning on this topic.
Chelsea was an Intelligence Analyst for the Army and used algorithms in the day to day duties of her job. She and I have been discussing algorithms, and their ethical implications, since the very first day we spoke on the phone, back in October 2015.
Chelsea recently published as a New York Times Op-Ed on the subject: The Dystopia We Signed Up For.
From the Op-Ed:
“The consequences of our being subjected to constant algorithmic scrutiny are often unclear… algorithms are already analyzing social media habits, determining credit worthiness, deciding which job candidates get called in for an interview and judging whether criminal defendants should be released on bail. Other machine-learning systems use automated facial analysis to detect and track emotions, or claim the ability to predict whether someone will become a criminal based only on their facial features. These systems leave no room for humanity, yet they define our daily lives.”
A few weeks later, in December, I went to the Human Rights Data Analysis Group (HRDAG) holiday party, and met HRDAG’s Executive Director, Megan Price. She explained a great deal to me about the predictive software used by the Chicago police, and how it was predicting crime in the wrong neighborhoods based on the biased data it was getting from meatspace. Meaning, the data itself was “good” in that it was accurate, but unfortunately, the actual less-than-desirable behavior by the Chicago PD was being used as a guide for sending officers out into the field. Basically the existing bad behavior of the Chicago PD was being used to assign future behavior.
This came as a revelation to me. Here we have a chance to stop the cycle of bad behavior, by using technology to predict where the next real crime may occur, but instead, we have chosen to memorialize the faulty techniques used in the past into software, to be used forever.
I have gradually come to understand that, although these algorithms are being used in all aspects of our lives, it is not often clear how or why they are working. Now, it has become clear that they can develop their own biases, based on the data they have been given to “learn” from. Often the origin of that “learning data” is not shared with the public.
I’m not saying that we have to understand how exactly every useful algorithm works; which I understand would be next to impossible, but I’m not sure a completely “black box” approach is best at least when the public, public data, and public safety are involved. (Thomas Hargrove’s Murder Accountability Project‘s “open” database is one example of a transparent approach that seems to be doing good things.)
There also appears to be a disconnect with law enforcement, while some precincts seem to be content to rely on on technology for direction, for better or worse, such as the predictive software used by the Chicago Police Department. In other situations, such Thomas Hargrove’s, “Murder Accountability Project” (featured in the article Murder He Calculated) technologists are having a hard time getting law enforcement to take these tools seriously. Even when these tools appear to have the potential to find killers, there appear to be numerous invisible hurdles in the way of any kind of a timely implementation. Even for these “life and death” cases, Hargrove has had a very hard time getting anyone to listen to him.
So, how do we convince law enforcement to do more with some data while we are, at the same time, concerned about the oversharing other forms of public data?
I find myself wondering what can even be done, if simple requests such as “make the NCIC database’s data for unsolved killings searchable” seem to be falling on deaf ears.
I am hoping to have some actual action items that can be followed up on in the months to come, as a result of this panel.
References:
1. The Dystopia We Signed Up For, Op-Ed by Chelsea Manning, New York Times, September 16, 2017. (Link goes to a free version not behind a paywall, at Op-Ed News)
2. Pitfalls of Predictive Policing, by Jessica Saunders for Rand Corporation, October 11, 2016. https://www.rand.org/blog/2016/10/pitfalls-of-predictive-policing.html
3. Predictions put into practice: a quasi-experimental evaluation of Chicago’s predictive policing pilot. by Jessica Saunders, Priscillia Hunt, John S. Hollywood, for the Journal of Experimental Criminology, August 12, 2016. https://link.springer.com/article/10.1007/s11292-016-9272-0
4. Murder He Calculated – by Robert Kolker, for Bloomberg.com, February 12th 2017.
5. Murder Accountability Project, founded by Thomas Hargrove. http://www.murderdata.org/
6. Secret Algorithms Are Deciding Criminal Trials and We’re Not Even Allowed to Test Their Accuracy – By Vera Eidelman, William J. Brennan Fellow, ACLU Speech, Privacy, and Technology Project, September 15, 2017. https://www.aclu.org/blog/privacy-technology/surveillance-technologies/secret-algorithms-are-deciding-criminal-trials-and
7. Machine Bias – There’s software used across the country to predict future criminals. And it’s biased against blacks. by Julia Angwin, Jeff Larson, Surya Mattu and Lauren Kirchner, ProPublica, May 23, 2016. https://www.propublica.org/article/machine-bias-risk-assessments-in-criminal-sentencing
8. Criminality Is Not A Nail – A new paper uses flawed methods to predict likely criminals based on their facial features. by Katherine Bailey for Medium.com, November 29, 2016. https://medium.com/backchannel/put-away-your-machine-learning-hammer-criminality-is-not-a-nail-1309c84bb899
Saturday, November 4, 2017
2:00 – 2:45 pm – Ethical Algorithms Panel – w/Q and A.
Kristian Lum (Human Rights Data Analysis Group – HRDAG) As the Lead Statistician at HRDAG, Kristian’s research focus has been on furthering HRDAG’s statistical methodology (population estimation or multiple systems estimation—with a particular emphasis on Bayesian methods and model averaging).
Caroline Sinders (Wikimedia Foundation) – Caroline uses machine learning to address online harassment at Wikimedia, and before that, she helped design and market IBM’s Watson. Caroline was also just named as one of Forbes’ “8 AI Designers You Need to Know.” Plus Special guests TBA